Vision-based Autonomous Car Racing Using Deep Imitative Reinforcement Learning
Abstract
Autonomous car racing is a challenging task in the robotic control area. Traditional modular methods for this task require accurate mapping, localization and planning, which makes them computationally inefficient and sensitive to environmental changes. Recently, deep-learning-based end-to-end systems have shown promising results for autonomous driving/racing. However, they are commonly implemented by supervised imitation learning (IL), which suffers from the distribution mismatch problem, or by reinforcement learning (RL), which requires a huge amount of risky interaction data. In this work, we present a general deep imitative reinforcement learning approach (DIRL) which successfully achieves agile autonomous racing using visual inputs. The driving knowledge is acquired from both IL and model-based RL, where the agent can learn from human teachers as well as self-improve by safely interacting with an offline world model. We validate our algorithm both in a high-fidelity driving simulation and on a real-world 1/20-scale RC-car with limited onboard computation. The evaluation results demonstrate that our method outperforms previous IL and RL methods in terms of sample efficiency and task performance.
System Architecture
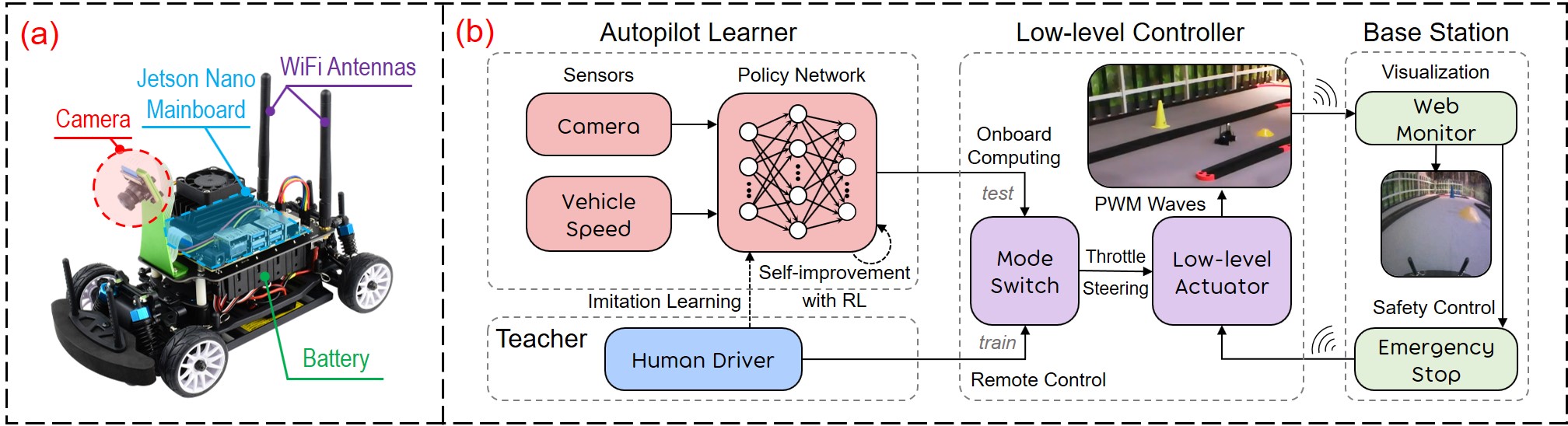
Video
Comparative Results
The bold font highlights the best results in each column.
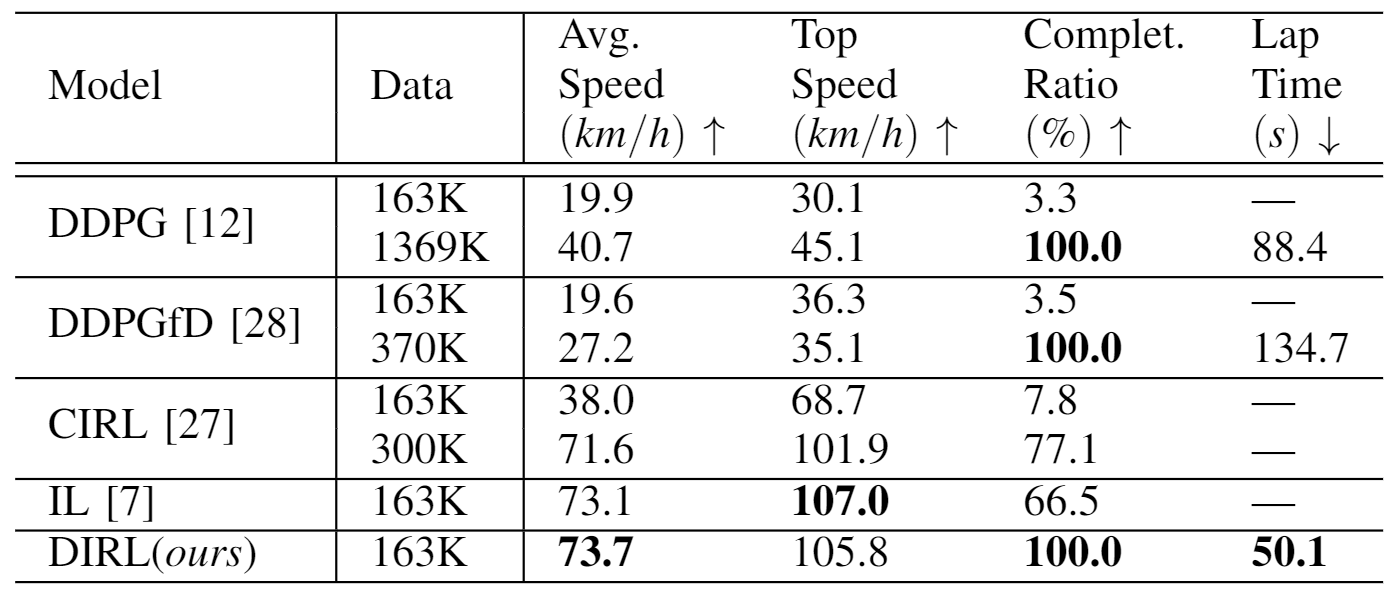
Overview of the Algorithm
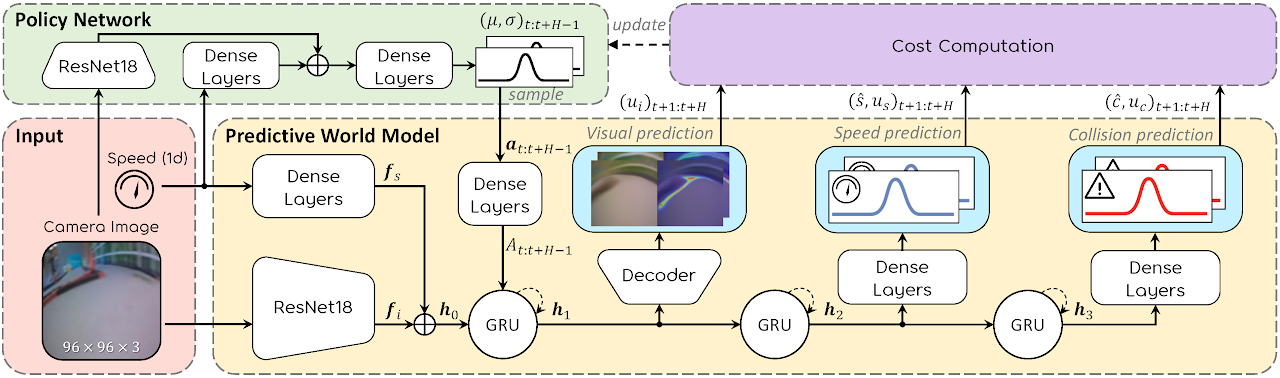